
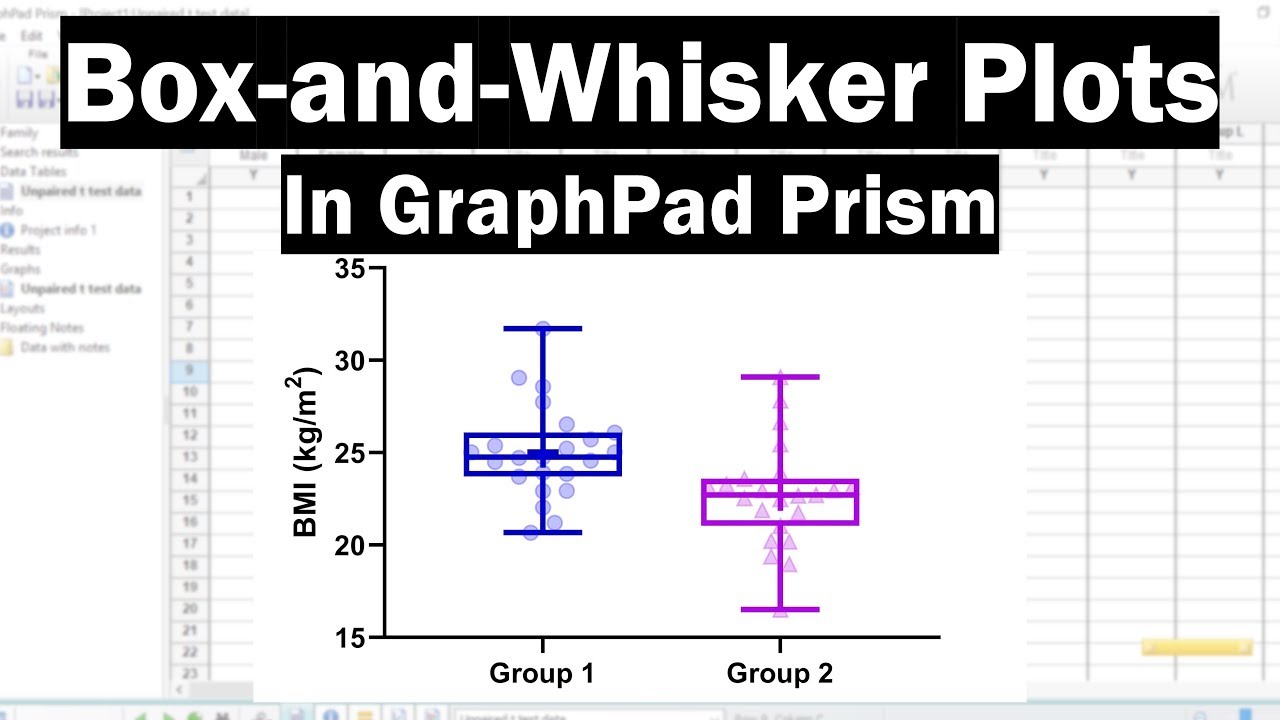
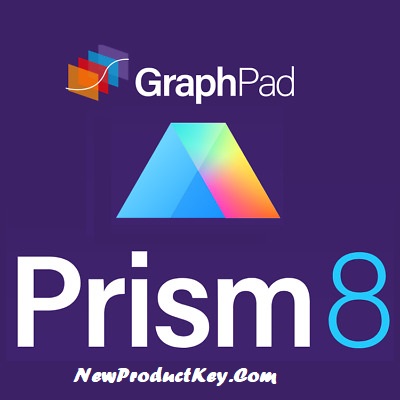
The study serves as a practical guide to help researchers who are not statisticians understand statistical tools more clearly, especially dose-response curve fitting using nonlinear regression. A review of popular fitting models using linear and nonlinear regression is given in. This non-iterative algorithm was implemented in a microcomputer and assessed using different biological and medical data. Nonlinear and non-iterative least square regression analysis was presented in for robust logistic curve fitting with detection of possible outliers. All of these demands can, without doubt, be applicable to large sets of data. To obtain proper curve fitting results, there is a need for automatic outlier handling, usage of predefined initial curves, and adaptive weighting for data points. However, due to the sensitivity of the method, the data quality and the initial guess, the curve fitting algorithm is unfortunately susceptible to becoming trapped in local minimums. This method belongs to the gradient-descent family. One of the widely used nonlinear curve fitting algorithms was introduced by Levenberg-Marquardt (LM). Several solutions - have been proposed to perform curve fitting. The quality of a dose-response curve fitting algorithm is a key element that should help in reducing false positive hits and increasing real compound hits. The overall process of fitting, rejecting outliers, and data point weighting of thousands to millions of curves can be a significant challenge. Performing large-scale curve fitting for the biological activity of a large compound library can lead to a great number of dose-response curves (DRCs) that require proper fitting. More recently, we applied the siRNA knockdown strategy for each gene from the genome and studied their effect on a given molecule producing a clear response on a cellular assay, to look for synergestic effects of a drug and target. At the Institut Pasteur Korea (IP-K), we have applied high content screening using small interfering RNA (siRNA), at the genome scale. However, HCS always comes with an important caveat: there is little or no a priori information on the compound’s target. HCS (also known as phenotypic screening) is a great tool to identify small molecules that alter the disease state of a cell based on measuring cellular phenotype. In recent years, the need for automatic data analysis has been amplified by the use of high content screening (HCS) techniques.
